Counting Elephants from the Sky: An Innovative Approach to Wildlife Population Monitoring
The motivation: Of all living mammals on Earth, 60% are livestock, 36% are humans, and a mere 4% are wild animals. Human civilization has caused a significant loss of wildlife and plant species, with 83% and 50% respectively declining in a relatively short time. The rate of species extinction is accelerating among both large and small animal species. The African bush elephant in particular has been deeply affected by this trend. According to a survey conducted in 18 African countries based on historical data, the population of African bush elephants (Loxodonta africana) decreased by an estimated 144,000 between 2007 and 2014, with the population shrinking by 8% per year continent-wide, primarily due to poaching. The ability to count African elephants automatically is critical to designing appropriate environmental policies and monitoring their populations in relation to biodiversity to maintain balance. However, manual counting methods are laborious, expensive, limited, and logistically challenging.
The method: An accurate and efficient method of monitoring and counting elephants is necessary to overcome these challenges. The use of satellite imagery and aerial photography has emerged as a promising solution, as it enables the coverage of vast areas in a short time. When aerial survey is complemented with computer vision and deep learning techniques, it has the potential to accelerate animal census and provide more accurate insight into elephant populations, allowing organizations to focus on elephant conservation. To accurately count African elephants from aerial photographs, a deep learning model was developed that takes an aerial image as input and generates a density map of the elephants’ locations. The density map is used to estimate the number of elephants accurately in the given image. The deep learning model was trained in a supervised manner using 2,074 aerial images of African bush elephants. These images were collected from various regions in South Africa, Botswana, and Namibia. The images were annotated with the precise location of each elephant by a human expert. By training the deep learning model on this diverse dataset, the counting accuracy of African elephants was significantly improved.
The results: To accurately count African elephants from aerial photographs, a deep learning model was trained exploiting a novel feature extractor technique. The prediction accuracy of the model was measured using the Root Mean Square Error (RMSE) between the predicted and actual animal count. The experimental results demonstrated that our model achieved high accuracy in counting African elephants, with an RMSE of 0.60, even in challenging conditions such as complex backgrounds, varying illumination, and heavy animal occlusion and overlapping.
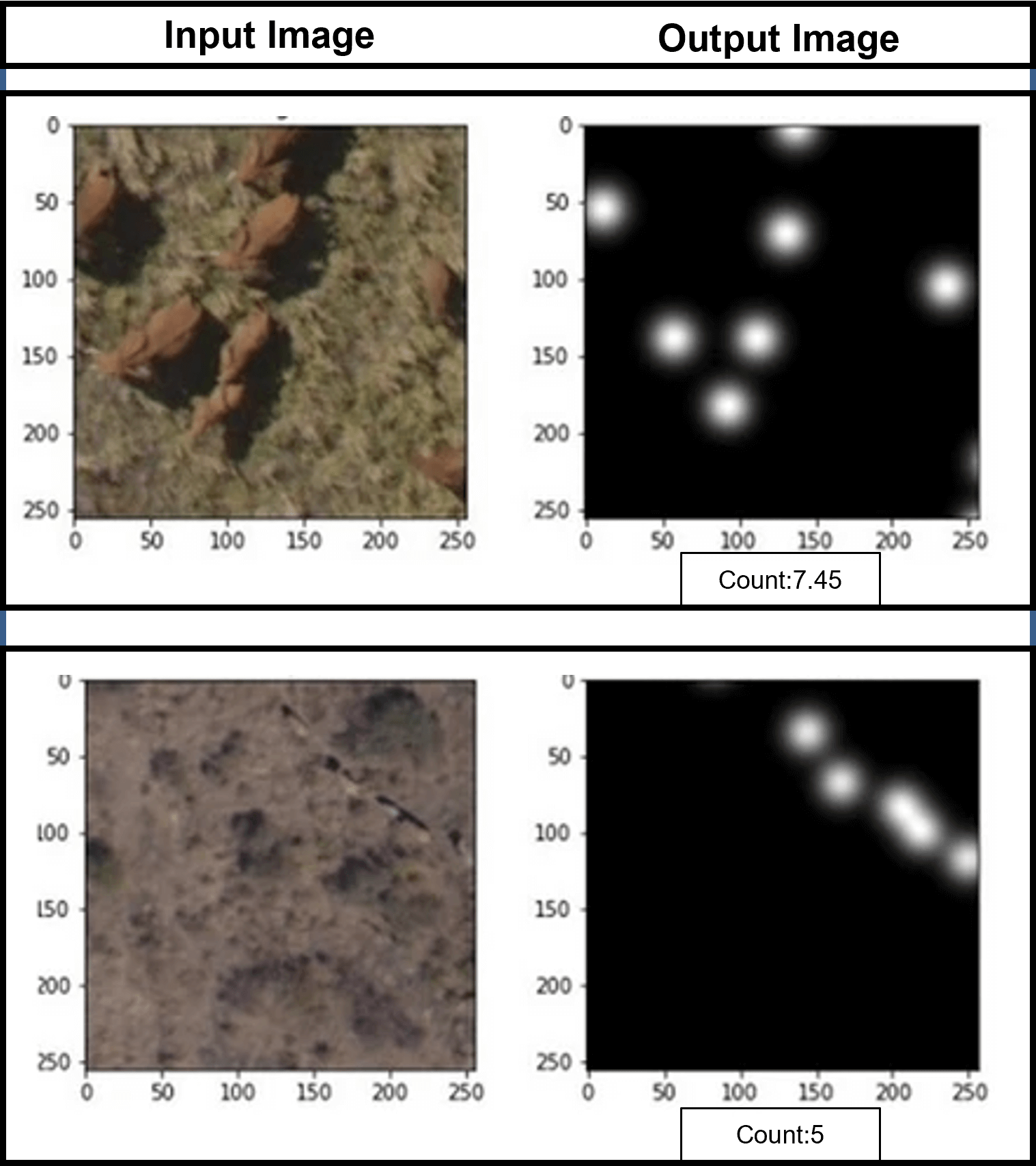
The importance: Aerial surveys of elephant populations are an essential tool for wildlife conservation, providing valuable information about elephant distribution, population size, and demographics. The use of unmanned aerial vehicles (UAVs) and deep learning algorithms can enable fast and accurate counting of elephants over large areas, while reducing the risk to human observers. Furthermore, deep learning models can be trained to detect elephants even in challenging environments, such as dense vegetation, forest canopies, or low light conditions. The benefits of accurate elephant counting through aerial images and deep learning extend beyond wildlife conservation. Elephant populations are important indicators of ecosystem health, and changes in their numbers and distribution can have cascading effects on the broader ecosystem. Accurate and timely monitoring of elephant populations can inform conservation policies and help mitigate conflicts between humans and elephants, such as crop raiding and habitat destruction. By combining aerial images and deep learning techniques, we can generate valuable insights into elephant populations and contribute to the ongoing effort to conserve these majestic animals and their habitats.
The Perspective: Monitoring wildlife populations is essential for conservation efforts, and new technologies like deep learning and aerial photography offer innovative solutions to this challenge. With the proposed counting method using aerial images and density maps, monitoring elephant populations can become more accurate and efficient. This advanced approach not only helps to protect these endangered animals but also offers valuable insights into their behavior, social structure, and habitat use. The significance and potential of elephant counting using density maps are paramount to elephant conservation efforts.
Chirag Padubidri, Andreas Kamilaris, Savvas Karatsiolis and Jacob Hamminga, Counting Sea Lions and Elephants from Aerial Photography using Deep Learning with Density Maps, In Animal Biotelemetry Journal, vol. 9, no. 27, August 2021. https://doi.org/10.1186/s40317-021-00247-x