Identifying invasive species in Japanese forests
The motivation: Invasive species are a threat to any ecosystem and can adversely overturn its balance which is key to the well-being and survival of its inhabitants. Invasive species can lead to the extinction of native plants and animals, destroy biodiversity, and reduce and alter wildlife habitat. Therefore, the spread of invasive species should be prevented to avoid huge negative effects on the environment, animal health, and safety and maintain healthy ecosystems.
The method: Black locust is an invasive tree species causing devastating problems to Japan’s mixed forests. We developed a Deep Learning model that identifies the specific tree species and maps its locations on aerial imagery collected by Unnamed Aerial Vehicles (UAVs)[1]. This could greatly help local authorities free the forests from specific tree species and regulate their infiltration into the ecosystem. The forest areas used for implementing the model span 2.5 sq. km and their locations and aerial images are shown in Figure 1.
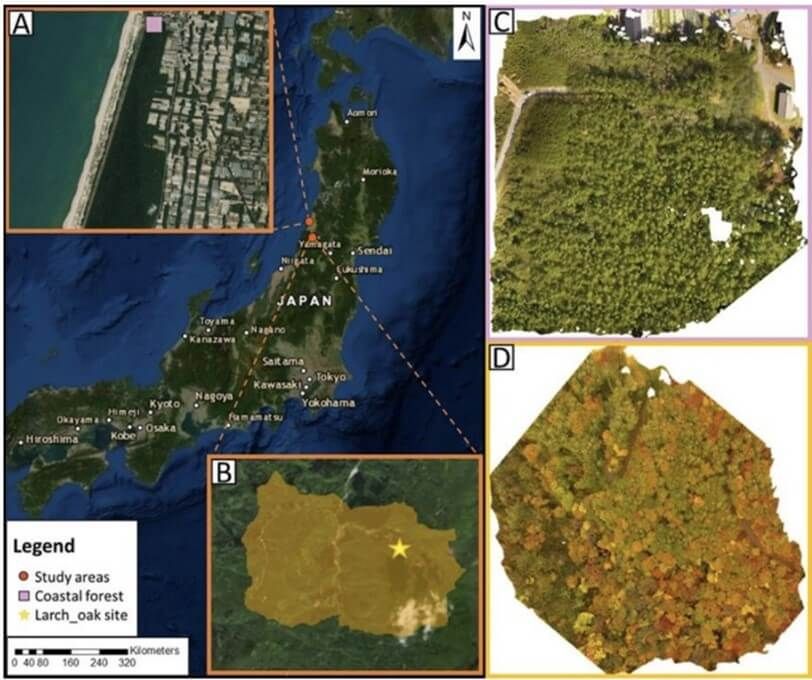
Figure 1. The location of the Japanese forests used in the study and their aerial images.
The results: The model identifies the Black Locusts with a precision of 63% and a recall of 98% which is on par with the state-of-the-art models that conduct invasive species detection. We also implemented another model identifying Japanese Larch trees inside a forest and we achieved a precision of 38% and a recall of 97%. The latter results are considered satisfactory considering that the Japanese Larch is a sub-alpine deciduous conifer, which makes its identification very hard during Autumn and Winter, periods during which it has no foliage. The model produces a segmentation map showing the location of the invasive tree that it detects. This map corresponds to the input aerial image which is useful for locating the trees as shown in Figure 2.

The perspective: This work shows the great potential of Deep Learning in identifying and locating invasive species in an aerial image and thus equipping policymakers and local authorities with a useful tool for dealing with species that don’t belong to the environment under focus. The methodology could be extended to other domains of remote sensing such as invasive animal or small plant species and is suitable for large-scale forestry management applications. Climate change risks and other relevant environmental risks posed on forests and wildlife in general due to the human factor could be mitigated with the use of AI-assisted large-scale monitoring.
[1] Sarah Kentsch, Savvas Karatsiolis, Andreas Kamilaris, Luca Tomhave and Maximo Larry Lopez Caceres, Identification of Tree Species in Japanese Forests based on Aerial Photography and Deep Learning, In Proc. of EnviroInfo, Nicosia, Cyprus, September 2020.